The State of AI in Cybersecurity
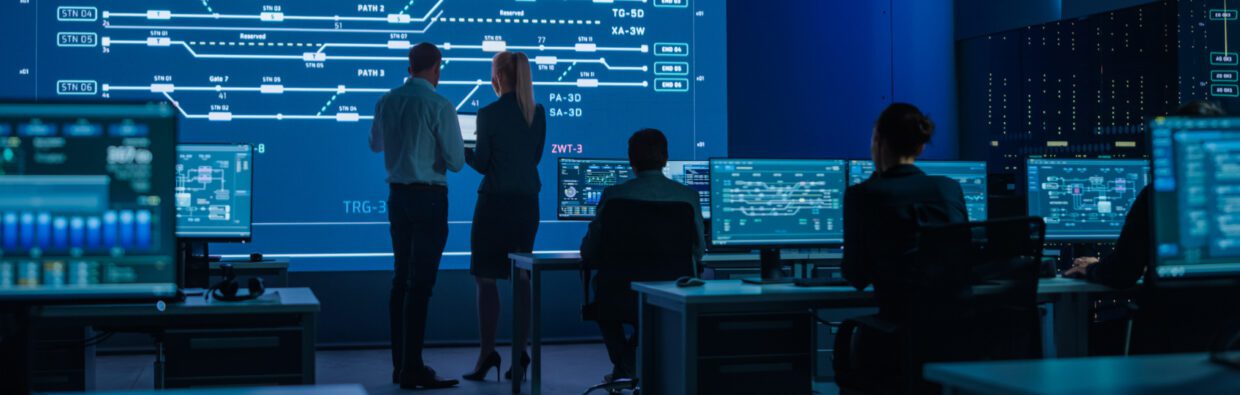
For years, cyberthreats have been detected by artificial intelligence (AI) — with machine learning as the main technique to implement artificial intelligence. Indeed, most of today’s security vendors, from small startups to large corporations, use AI in their products to detect malicious activity.
AI delivers value by finding malicious files, emails or websites, or by detecting unusual user or system behavior. Notably, Microsoft uses AI extensively in its Defender, Sentinel, and other security tools to successfully detect attacks.
However, while the success stories are numerous and AI seems to have reached a critical mass in the public consciousness, there are also examples where AI hasn’t lived up to promises and lofty expectations. AI systems often have the reputation of generating excessive numbers of false positives, and the black-box nature of many AI systems raises concerns with many experts. With exaggerated expectations that haven’t lived up to reality, many security experts have grown skeptical towards AI in cybersecurity.
Yet, recent developments, mostly triggered by the hype around ChatGPT, have put AI squarely in the spotlight. And with this, many people wonder how these recent technologies will be put to use in cybersecurity. Will the visions and prototypes of fully automated, intelligent security systems become reality this time? Will AI be the key to put cyber criminals out of business? Or will it take a darker turn and prove more valuable to the attackers than the defenders?
With these questions in mind, we discuss three exciting topics around AI in cybersecurity:
- The impact of generative AI like GPT on security operations
- Innovative use cases beyond detection of malicious activity
- The inevitable prospect of attackers using AI
Generative AI: What has changed with the rise of GPT?
With the launch of ChatGPT, AI had its “iPhone moment.” GPT stands for “generative pre-trained transformer,” and its ability to not only produce text, but to produce chain-of-thought, and seemingly human-like reasoning about a subject was an eye-opening realization. This realization has stirred new hopes about the potential — but also fear of new risks — of the technology. Not the least in the security field.
Large Language Models (LLMs) as force multipliers
To this end, Microsoft has announced a private preview of the Security Copilot, connecting GPT to security tools like Defender, Sentinel, InTune, and more. Other vendors like Google and CrowdStrike are following quickly. The promise of such “copilot” and assistant tools is to super-charge the human security analysts by giving them a natural language interface to data needed to be more efficient at threat hunting, incident investigation, malware analysis, and other security activities.
While currently valuable mostly as force multipliers for human analysts, the reasoning and planning capabilities of large language models (LLMs) are inspiring scenarios that go a step further and remove the human from these tasks completely: positioning the LLM as the automated security analysts. While such use cases may become possible in the future, at the current state of the technology the human must remain in the driver’s seat and make the final call about crucial security decisions.
Generating training data for detection systems
Besides supporting human security analysts, we will see other applications of generative AI soon. For example, LLMs can generate artificial phishing attacks to better train phishing detectors, or to use for awareness training. Similarly, the code generation capabilities of LLMs can be used to generate malware variants to better train malware detection. Creating artificial network data for Network Detection and Response (NDR) or anomaly detectors, artificial user behavior for user and entity behavioral analytics (UEBA) systems, the possibilities are almost endless.
Improving communication
Another class of applications will focus on communication between security people and toward customers and other stakeholders. The ability to quickly produce reports, to summarize complex events concisely —and even produce slides to present to management — will support security professionals in communicating efficiently and effectively.
These are just a few examples of the immense potential of generative AI in cybersecurity. However, with all the hype around the topic, it’s important to keep in mind two key points:
The LLM needs to be connected to contextual data about the specific environment.
To mitigate the confabulations and hallucinations that are typical with LLMs (where the LLM generates a response without any basis in fact), the human must remain in the driver’s seat — with the AI acting as a force multiplier.
Conclusion
Though at times failing to live up to its hype, AI has nevertheless changed the way cybersecurity is able to respond to threats and protect data. But we are at an inflection point, and the existing use cases are just the tip of the iceberg.
Watch for the second of this two-part series, where we’ll explore new and exciting ways that AI can move beyond threat detection to proactively help harden environments and optimize defenses.